Three practical steps towards implementing effective data governance to improve data security and achieve interoperability
Data interoperability is the ability of different systems and organizations to work together to share knowledge and collaborate, and it’s essential for effective decision-making and sustainable development. Yet, a recent SurveyCTO user survey found that only 28% of respondents from development organizations were familiar with the term “interoperability” in relation to data collection and management. Of those respondents, just 39% had designed their data processes to be interoperable with other systems.
These statistics highlight a pressing need for greater focus on interoperability within the development sector. But changing data collection and management processes to be better-suited towards greater interoperability can be daunting! If you’re thinking of ways to get your data processes to be more interoperable, it can be a good idea to work towards individual components of interoperability and treat them as building blocks towards your overall goal.
Data governance: A building block for interoperability
One key factor in achieving data interoperability? Having robust data governance and accountability frameworks for your organization.
Effective data governance involves a strategy that defines priorities, procedures, and guidelines that an organization follows to manage its data assets responsibly and effectively. It supports interoperability by establishing clear guidelines and standards for data management, which promotes accountability, consistency, and trust in the data being shared and used across different platforms and stakeholders. This is essential for maintaining data integrity and facilitating interoperability. Without these frameworks, organizations risk inefficiencies and missed opportunities in their missions to drive sustainable development, as noted by the Global Partnership for Sustainable Development Data:
The demand for useful and usable information – produced from contextualised, joined-up, interoperable data – is the key driver in the creation of the sustainable data infrastructures that will ensure that all people can be counted repeatedly and cost-effectively so that no one is left behind.
Tom Ordell and Bill Anderson, Global Partnership for Sustainable Development Data
Why data governance matters in interoperability
Data governance involves managing data availability, usability, integrity, and security to ensure that data across different systems, departments, and even countries is consistent, trustworthy, and appropriately used. Many organizations, including the United Nations (UN), and NetHope, emphasize the importance of data governance.
Without robust data governance, development organizations can face challenges such as data silos, inconsistent data formats, and security vulnerabilities. All of these challenges hinder effective data sharing–and also decrease general data quality. In addition, data governance plays a crucial role in achieving interoperability by bringing clarity in strategic direction, aligning data collection and analysis with organizational objectives, and tracking adherence to data governance standards.
Here’s how NetHope put it in their Data Governance Toolkit:
Data governance is the necessary cornerstone to ensuring that data is consistent, trustworthy and doesn't get misused.
NetHope Data Governance Toolkit
Types of data governance frameworks
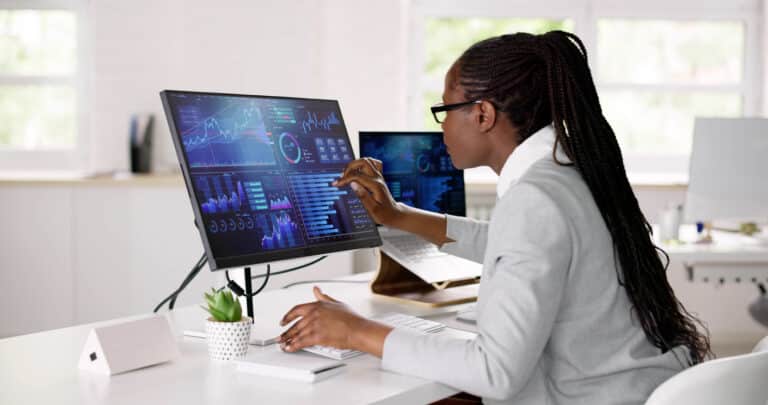
A data governance framework or template is a structured set of principles and processes that dictate how data is collected, stored, and utilized within an organization. Organizations need to choose the approach that aligns best with their objectives and operational needs.
Different frameworks cater to diverse organizational requirements and structures. For instance, the UN describes five frameworks ranging from centralized to decentralized approaches. Here, we present a simplified three-part framework, as outlined by Claravine, a data standards company, that effectively encapsulates the core types of data governance models an organization might use:
Centralized data governance
In a centralized data governance model, a single entity or individual is responsible for making decisions and setting the direction for the entire data governance program. This model is beneficial for organizations that need strong control over data quality and security, as it ensures consistent data management practices across the board. However, it can also lead to bureaucracy and may not address the specific needs of different departments within an organization.
Decentralized data governance
The decentralized model involves multiple departments or functional areas within an organization managing their own data sets and governance processes. This approach allows for more tailored data management practices that meet the specific needs of each department. However, it can result in inconsistent data standards and duplication of data, which can complicate efforts to achieve interoperability.
Hybrid data governance
A hybrid or federated model combines elements of both centralized and decentralized approaches. It provides a centralized framework and best practices for data governance, but allows individual departments or units to manage their own data and develop standards that best suit their specific needs. This model offers the benefits of both approaches, promoting consistency while allowing for flexibility and innovation.
Governance and accountability
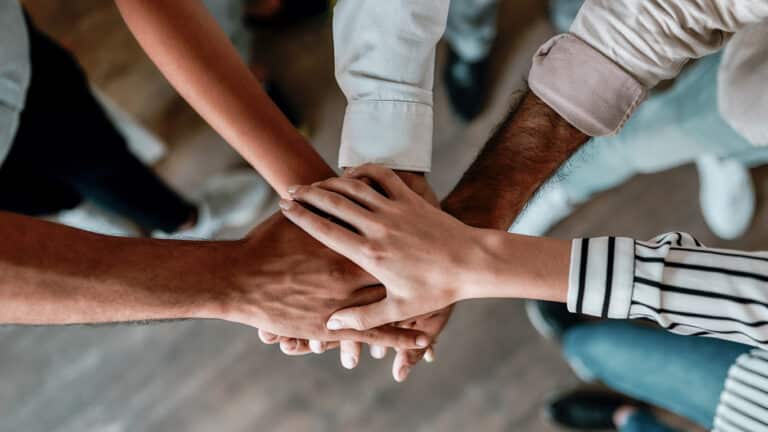
Another foundational element closely related to good governance is accountability. According to the United Nations, embedding data interoperability as a guiding principle requires meticulous planning of governance mechanisms, with a strong emphasis on oversight and accountability. Accountability ensures that data is managed consistently and transparently across its lifecycle, enabling seamless integration and use across different systems and departments.
Accountability in data governance is not just about adhering to standards and regulations; it’s about fostering a culture of responsibility where every stakeholder understands their role in maintaining data integrity. This cultural shift is essential for creating an environment where data can be trusted and effectively shared, thereby enhancing interoperability.
Roles and responsibilities in achieving accountability
In a strong data governance framework, roles and responsibilities are clearly defined to ensure accountability at every level of data management. Key roles such as data governance officers, stewards, custodians, and executive sponsors each have specific duties. Depending on the role, these responsibilities may include setting strategic direction, ensuring data accuracy and security, or making sure that data governance initiatives are properly resourced and prioritized.
Approaches to oversight for accountability
Oversight and accountability in data governance vary depending on an organization’s size, structure, and resources. The common approaches mirror those of general data governance, as they include centralized oversight, where a central council ensures consistency across large organizations; decentralized oversight, where individual departments manage governance independently in smaller or decentralized setups; and hybrid oversight, which blends centralized control with departmental autonomy to balance accountability and flexibility.
How to achieve better data governance in your organization
Once you’ve determined the data governance framework and accountability approaches that best suits your organization, it’s time to take actionable steps to ensure successful implementation. Here’s a comprehensive guide to help you navigate the process:
1. Create and document your data governance policy
The foundation of effective data governance starts with a well-crafted policy. This policy should outline the principles, roles, and processes that will guide your organization in managing its data assets. Here’s how to get started:
- Develop a data governance framework: Before drafting your policy, create a robust framework. This involves completing a needs assessment to identify priority areas, assessing data risks, and determining data ownership.
- Needs assessment: Identify where data is exchanged within your business units and how it is managed. This assessment should also address risks such as data quality, security, and access issues.
- Stakeholder matrix: Develop a stakeholder matrix to identify key stakeholders and secure their buy-in. This matrix will help you understand who needs to be involved and how to communicate with them effectively.
- Measure key performance indicators (KPIs): Define KPIs, such as number of projects where data governance is enforced or policies defined, to measure the success of your data governance implementation.
2. Identify roles and responsibilities
Clearly defining roles and responsibilities related to data within your organization is crucial for ensuring accountability and the smooth operation of your data governance policy. First steps:
- Assign roles: Identify and assign roles. These can include Data Governance Officers (DGOs), data stewards, and data custodians. These roles are responsible for various aspects of data management, including quality, security, and compliance.
- Define responsibilities: Ensure that each role has a clear set of responsibilities. For instance, DGOs might oversee the entire data governance program, while data stewards manage specific data sets and ensure compliance with governance policies.
- Training and support: Provide training and ongoing support to those in data governance roles. This ensures they are well-equipped to handle their responsibilities and can adapt to new challenges as they arise.
3. Have a process for ongoing improvement
Data governance is not a one-time project but a continuous process that evolves with your organization. To maintain the effectiveness of your data governance policy, establish processes for ongoing support and improvement:
- Regular audits and reviews: Conduct regular data audits and quality assessments to ensure compliance with governance policies. Use these audits to identify areas for improvement and update policies as needed.
- Continuous improvement: Develop a plan to continuously nurture and grow your data governance practices. This includes updating your framework and policies to reflect new regulatory requirements and business needs.
- Feedback mechanisms: Implement feedback mechanisms, whether that’s periodic meetings, surveys, or other regular practices to gather input from stakeholders at all levels. This helps in identifying practical issues and refining governance practices to better suit the organization.
4. Implement your data governance plan
With your policy documented and roles defined, the next step is to put your plan into action:
- Communication plan: Develop a communication plan to ensure that all stakeholders are aware of the data governance policy and their roles within it. Regular updates and training sessions can help maintain engagement and compliance.
- Technology evaluation: Continuously evaluate your technology stack to ensure you have the tools you need to support your data governance needs. This includes tools for metadata management, data cataloging, and data security.
- Monitor and measure: Use the KPIs defined in your data strategy to monitor the progress of your data governance implementation. Regularly review these metrics to ensure that your data governance practices are delivering the desired outcomes.
Further resources for data governance
We know data governance can seem challenging to those just getting started! To help deepen your knowledge, we’ve put together some additional resources for you to explore:
- Using the data lifecycle to manage data responsibly (Oxfam): This guide focuses on the responsible management of program data throughout its lifecycle, with a focus on humanitarian crises, while offering principles and tools applicable to broader contexts.
- Data governance toolkit (Nethope): This toolkit guides non-profit organizations in implementing data governance by providing best practices, templates, and a framework that aligns with evolving IT landscapes and program deliverables.
- Accountable data governance (data4sdgs.org): This website provides a number of articles and resources, including case studies, around data governance.
- Data governance frameworks: The cornerstone of data-driven enterprises (ClaraVine): This article explains what a data governance framework is, its core elements, operational models, and best practices.
- Data management, governance, and interoperability (United Nations): Within this longer guide on data interoperability, this chapter discusses the critical importance of data management and governance, emphasizing the need for strategic oversight, accountability, and effective frameworks across an organization’s data lifecycle.
- We need to solve real-world problems in a joined-up way (data4sdgs.org): This article discusses the Joined-Up Data Standards (JUDS) project, which aims to enhance data interoperability for sustainable development by fostering international collaboration.